You Can Teach an Old Dog New Tricks - Rank Fusion applied to Coordination Level Matching for Ranking in Systematic Reviews
Authors:
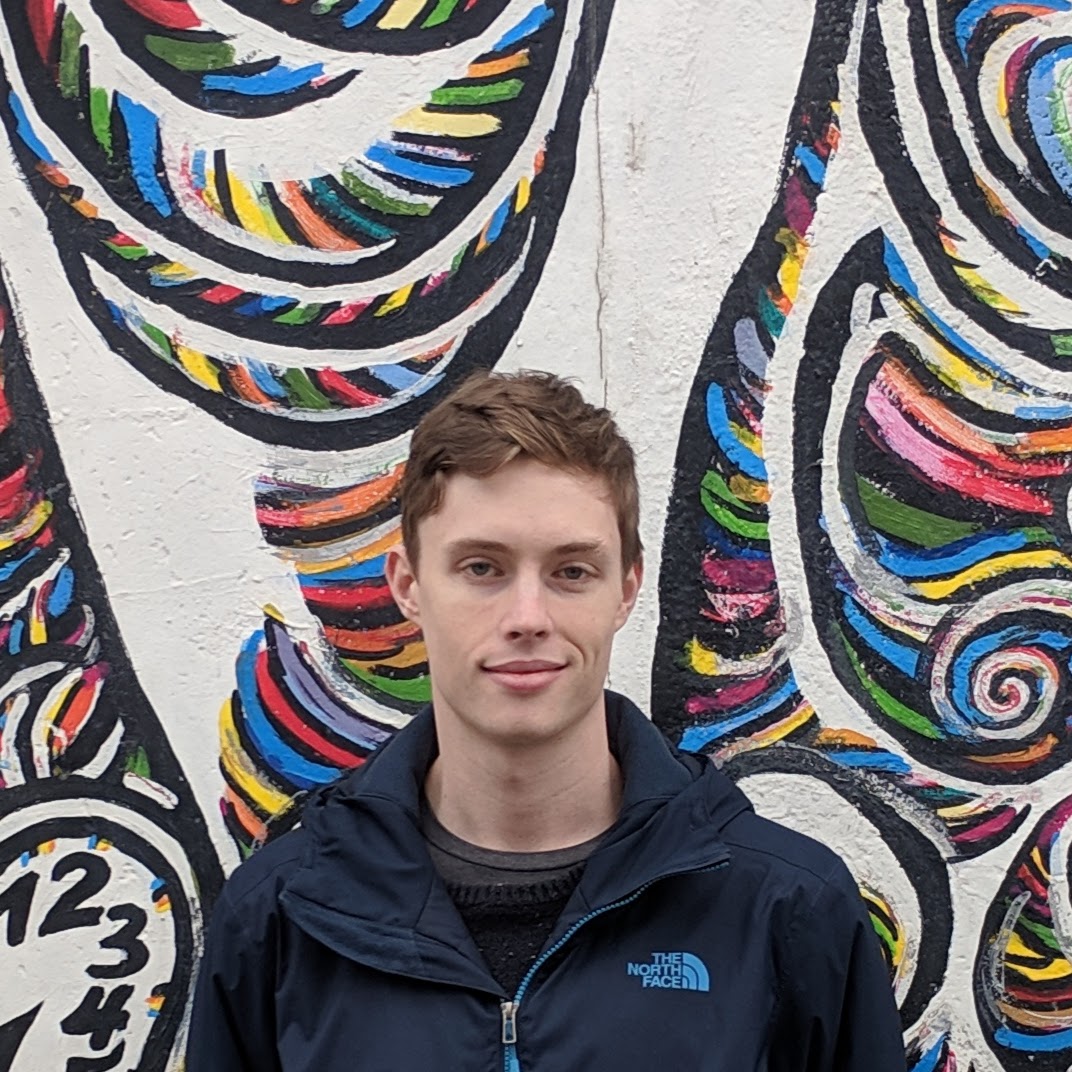
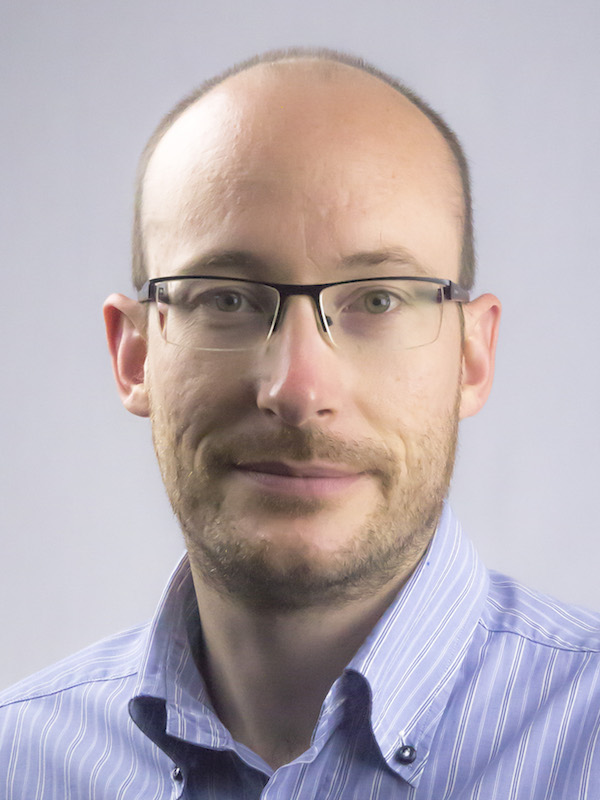
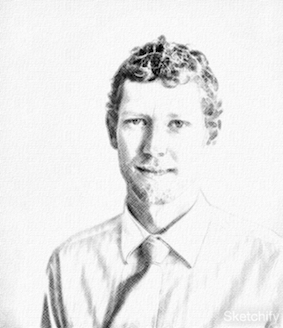
Coordination level matching is a ranking method originally proposed to rank documents given Boolean queries that is now several decades old. Rank fusion is a relatively recent method for combining runs from multiple systems into a single ranking, and has been shown to significantly improve the ranking. This paper presents a novel extension to coordination level matching, by applying rank fusion to each sub-clause of a Boolean query. We show that, for the tasks of systematic review screening prioritisation and stopping estimation, our method significantly outperforms the state-of-the-art learning to rank and bag-of-words-based systems for this domain. Our fully automatic, unsupervised method has (i) the potential for significant real-world cost savings (ii) does not rely on any intervention from the user, and (iii) is significantly better at ranking documents given only a Boolean query in the context of systematic reviews when compared to other approaches.